The evolution of OCR to intelligent AI
OCR (Optical Character Recognition) is a technology that transforms information from documents or images into digital text. It has been continuously developed from its inception to the era of intelligent AI. Today, we will take you on a journey through the evolution of OCR, from its beginnings to the latest advancements.
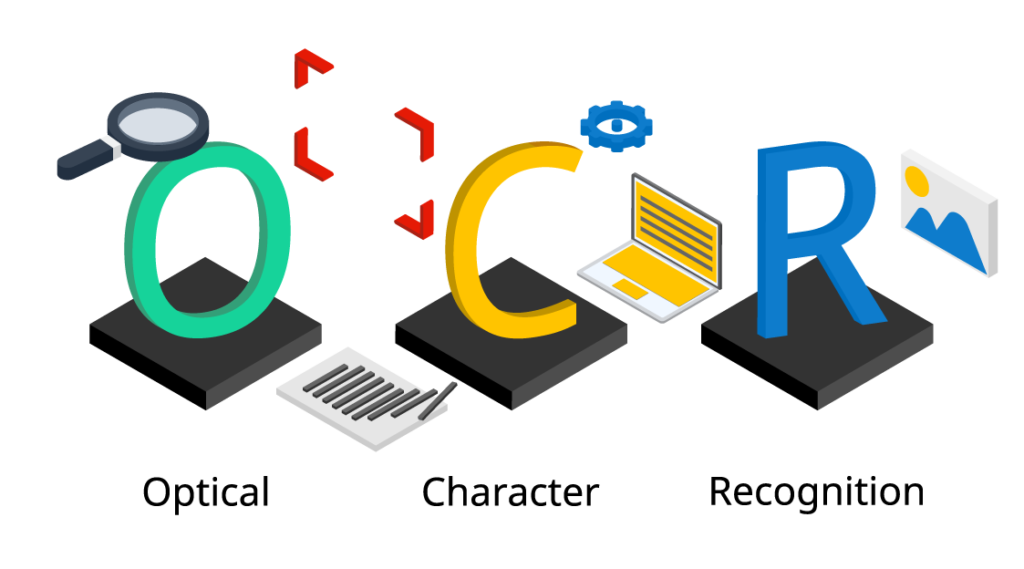
https://runsystem.net/en/2024/03/26/ocr-technology-helps-businesses-save-time/
1. The Beginning: Basic Character Reading
- The Pre-Computer Era (1920-1950)
Going back to the era before the advent of computers, OCR (Optical Character Recognition) technology began as an effort to develop tools that could assist humans in reading information from text on paper or other materials. It was particularly aimed at supporting visually impaired individuals and improving efficiency in data management processes. The key developments included:
Text-to-Sound Conversion (Optophone)
The Optophone was one of the earliest tools that marked the beginning of OCR technology. It was designed by Edmund Fournier d'Albe, a British scientist, in 1920. This device was developed to convert text into sound by using light reflected from characters on a surface. Although the Optophone could not recognize characters, it could produce distinct sounds corresponding to the shapes of letters. However, the device was limited by its speed and was suitable only for specific use cases.
Development of Opto-Mechanical Technology
In the late 1930s, researchers began utilizing photoelectric cells to develop systems capable of detecting and recognizing character patterns. This concept became a fundamental foundation for OCR technology, with designs enabling machines to "match" scanned characters to pre-stored character patterns.
Character Reading for Assisting the Disabled
Emanuel Goldberg, a German scientist, developed a device capable of reading characters and converting the information into Morse code. This was an early prototype that integrated character recognition technology with data conversion, enabling effective applications in communication.
The First Patent for an OCR System
In 1949, David H. Shepard, an American inventor, developed the world’s first OCR system and obtained its first patent. This machine was designed to convert text from standard printed characters into a format that machines could process. Its functionality was fundamentally based on the principle of pattern matching.
- The Emergence of Commercial OCR (1960-1980)
This period is considered the era when OCR (Optical Character Recognition) technology began to be seriously implemented in commercial applications. It was developed to meet the needs of organizations and industries aiming to reduce workloads through automation. OCR in this era saw advancements in various areas, including the support for a wider range of characters, increased speed, and improved accuracy in data recognition. Key developments during this time include:
Development of Document Processing Technology
During this period, OCR was designed to support the processing of data from printed documents such as bank checks, receipts, and purchase orders. It relied on key technologies like light sensors, which helped detect variations in color and ink density, allowing for more accurate character recognition on plain paper. Additionally, the OCR system continued to use the principle of comparing scanned characters with pre-defined character templates (Template Matching) to enhance data recognition efficiency.
Creation of OCR-A and OCR-B Fonts
OCR-A was developed in 1966 by the American National Standards Institute (ANSI) and was designed to be machine-readable. The characters feature clear, angular shapes that are simple and easy for machines to process.
OCR-B was designed by Adrian Frutiger to balance both aesthetic appeal and ease of machine readability. This font became widely popular in banks and financial transactions.
OCR in the Banking Industry (MICR)
Banks began adopting Magnetic Ink Character Recognition (MICR) systems, which can read characters printed with magnetic ink. MICR enables OCR to accurately read numbers on checks or financial documents printed with special fonts, such as E-13B.
Widespread Launch of Commercial OCR
In the 1960s, RCA developed and launched the first OCR machine for organizational use, capable of scanning documents and automatically extracting text. Later, in the 1970s, IBM developed a commercial OCR system that could read a variety of fonts, which was adopted by businesses and government agencies, such as for scanning tax documents.
Applications of OCR in Various Industries
OCR began to be used to read account numbers on checks and process financial documents in the banking and finance industries. It was also applied to scan addresses and postal codes in the mail, as well as to scan and store data from important documents in government agencies, such as birth certificates.
2. The Computer Era: Statistical Learning (1980-2000)
- Basic Applications of Machine Learning
Between 1980 and 2000, OCR technology entered a new era that began to rely on Machine Learning (ML) and Statistical Learning to enhance character recognition and support the processing of more complex data. The use of ML during this period marked a significant turning point, transforming OCR from a pattern-matching approach to learning from real data. Key developments during this time include:
The Shift from Template Matching to Machine Learning
Traditional OCR systems used pattern matching with predefined templates, which had limitations in handling a wide variety of fonts, blurry characters, or low-quality documents. However, with the use of Machine Learning, OCR systems can learn and adapt to new character patterns without the need for predefined templates.
Application of Neural Networks
Early Neural Networks, such as the Perceptron, were used to distinguish the characteristics of characters, such as straight lines, curves, and angles. During this era, the model learned the unique features of each character through a training process using large datasets, enabling it to handle a wide variety of fonts and languages, and improving its ability to recognize characters even in cases of unclear images.
Hidden Markov Models (HMMs)
HMMs were used in OCR to recognize sequences of characters by considering the relationships between each character. This allowed OCR not only to identify individual characters but also to correctly understand the structure of words or text within context.
3. The Revolution with AI and Deep Learning (2000-Present)
Since 2000, OCR has advanced rapidly with the revolution of AI and Deep Learning technologies. The integration of AI models has significantly enhanced OCR capabilities, from recognizing characters in complex formats to understanding the context of text in multiple languages. Key developments include:
- Using Deep Learning to Improve Accuracy
Convolutional Neural Networks (CNNs)
Convolutional Neural Networks (CNNs) are used in image processing to extract complex features of characters, such as curves or textures, which helps improve the accuracy of OCR, especially in documents with low quality.
Recurrent Neural Networks (RNNs)
Long Short-Term Memory (LSTM) models enable OCR to more effectively recognize characters that are related in sequence, improving its performance in text recognition.
Natural Language Processing (NLP)
Integrating AI and NLP with OCR enables the system to better understand the context of the text, such as analyzing the content in legal documents.
Handwriting Recognition
AI Handwriting Recognition technology has been developed to recognize handwritten text in various formats.
- Cloud-based OCR and real-time processing
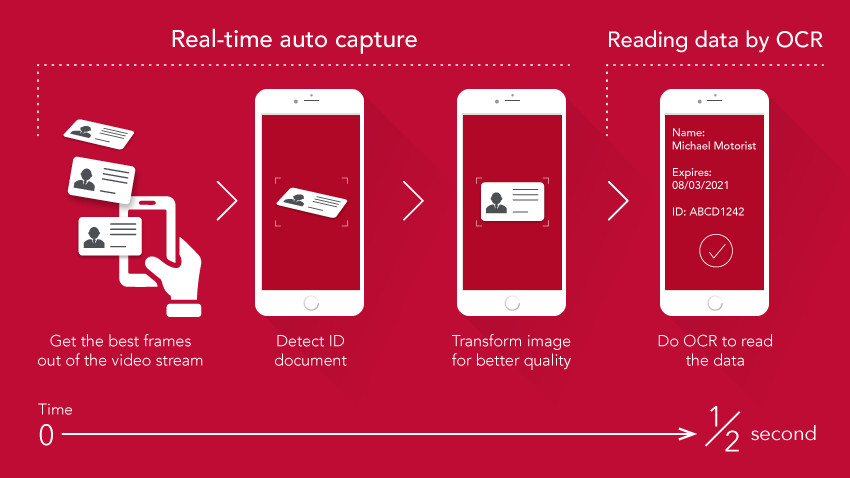
https://www.linkedin.com/pulse/real-time-auto-capture-user-friendly-approach-mobile-izet-%C5%BEdralovi%C4%87
Real-Time OCR
Currently, OCR can recognize text in videos and images in real-time and is used in various applications, such as Google Translate, which can instantly translate text from signs.
4. The impact of OCR in the AI era
OCR (Optical Character Recognition) technology, integrated with AI, has transformed workflows across various sectors, from business and education to daily life. Advances in accuracy, speed, and the ability to understand deep insights have led to significant impacts in multiple dimensions, with key developments as follows:
- Enhancing efficiency in the business sector
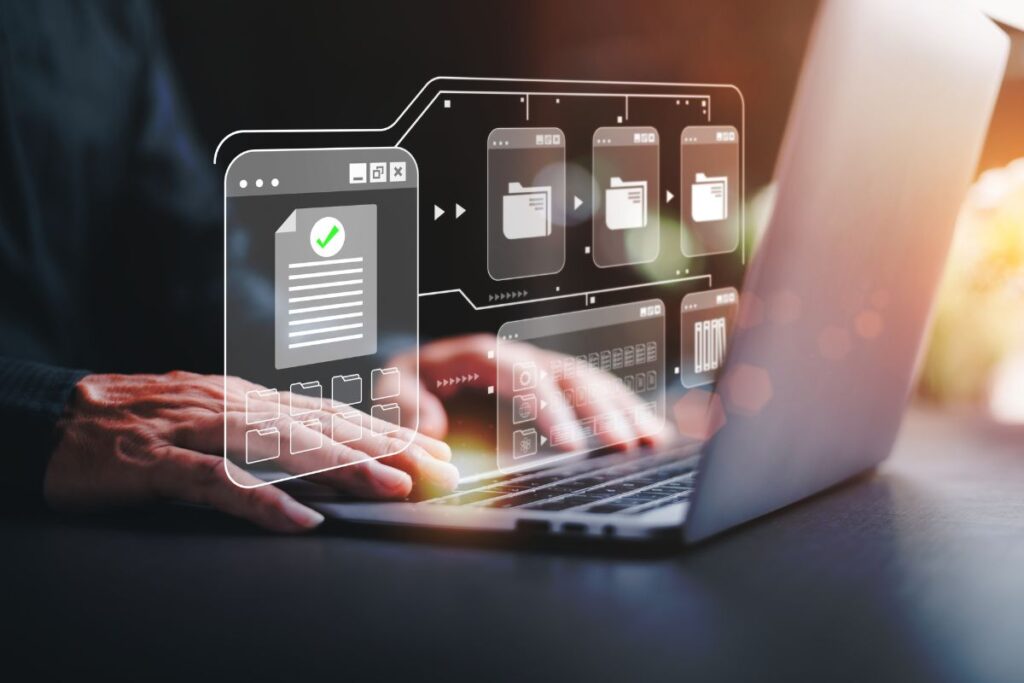
https://aigencorp.com/4-ocr-business-problems/
Document processing
OCR automatically reads information from invoices, contracts, and bills, reducing the time spent on manual data entry.
Big Data management
OCR can extract data from vast documents for in-depth analysis and support real-time business decision-making.
- Advancements in education and access to knowledge
Digitizing educational documents
OCR helps scan old books and articles to make them available in online formats and supports text search within digital documents.
Assisting visually impaired individuals
Integrating OCR with Text-to-Speech (TTS) helps convert text in documents into speech, enabling visually impaired individuals to access information.
5. The future of OCR
In the future, OCR technology will be able to learn from new data automatically, without the need for additional training, by using Reinforcement Learning and Active Learning. This will allow OCR to improve accuracy when handling diverse data.
- Supporting more complex data
In the future, OCR will be able to handle more complex data, such as recognizing messy handwriting or supporting multiple font types within the same document, as well as extracting information from tables, graphs, photographs, or maps.
- Enhancing security and data protection (Secure OCR)
In the future, OCR will emphasize data security, utilizing data encryption and local processing to reduce the risk of data leakage.